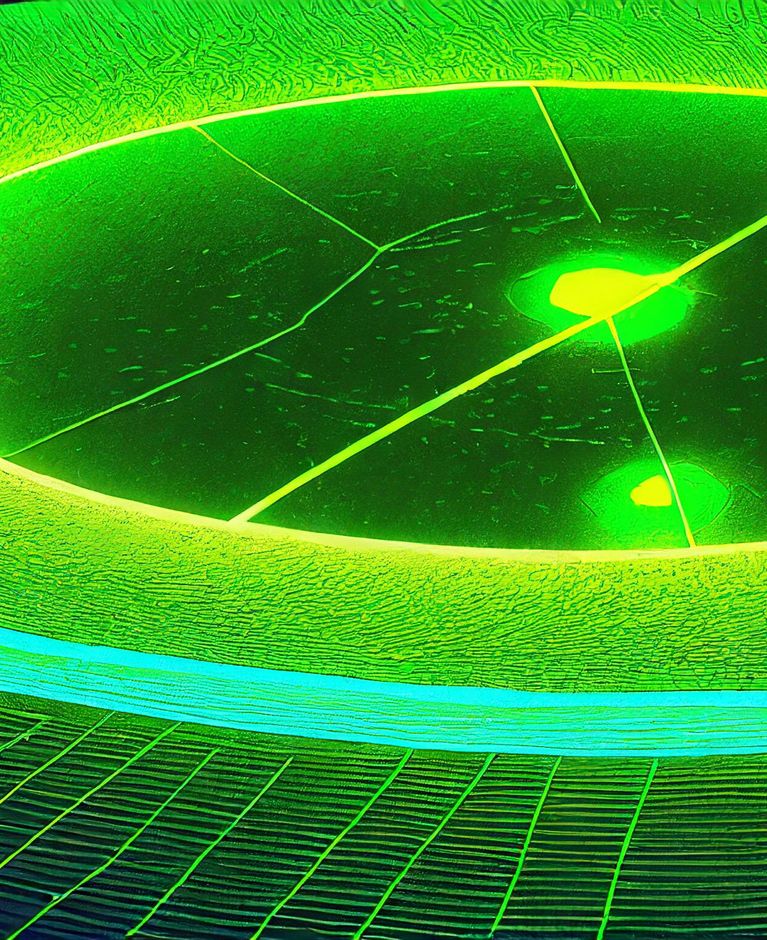
Sensing cutaneous manifestations of cardiometabolic diseases
Skin microangiopathy has been associated with diabetes. Here we show that skin-microangiopathy phenotypes in humans can be correlated with diabetes stage via morphophysiological cutaneous features extracted from raster-scan optoacoustic mesoscopy (RSOM) images of skin on the leg. We obtained 199 RSOM images from 115 participants (40 healthy and 75 with diabetes), and used machine learning to segment skin layers and microvasculature to identify clinically explainable features pertaining to different depths and scales of detail that provided the highest predictive power. Features in the dermal layer at the scale of detail of 0.1–1 mm (such as the number of junction-to-junction branches) were highly sensitive to diabetes stage. A ‘microangiopathy score’ compiling the 32 most-relevant features predicted the presence of diabetes with an area under the receiver operating characteristic curve of 0.84. The analysis of morphophysiological cutaneous features via RSOM may allow for the discovery of diabetes biomarkers in the skin and for the monitoring of diabetes status.
More details:
Dermal features derived from optoacoustic tomograms via machine learning correlate microangiopathy phenotypes with diabetes stage, Karlas, A., Katsouli, N., Fasoula, NA. et al. Dermal features derived from optoacoustic tomograms via machine learning correlate microangiopathy phenotypes with diabetes stage. Nat. Biomed. Eng 7, 1667–1682 (2023). doi.org/10.1038/s41551-023-01151-w
Contact
Vasilis Ntziachristos
Helmholtz Munich
E-Mail